UAD Unsupervised Data Augmentation
本文得出的结论是,用在有标签数据上增强效果好的方法,用在无标签数据上也好在数据增广的时候,假如刚好截取了没有猫的背景部分, 生成
本文得出的结论是,用在有标签数据上增强效果好的方法,用在无标签数据上也好
在数据增广的时候,假如刚好截取了没有猫的背景部分, 生成新的训练数据 x'。此时 x' 的标签与 x 不同。强迫神经网络对 x 和 x' 都预测为猫, 毫无意义。UDA 紧抓这一点,使用智能增广技术,AutoAugment,使得数据增广不破坏标签.
Training Signal Annealing技术,来防止有标签数据过拟合。具体来说,如果一个有标签数据的预测概率超过了当前阈值,那么在这次计算loss时就不算它的了(因为它已经表现很好了,再训练也不过是过拟合而已)。
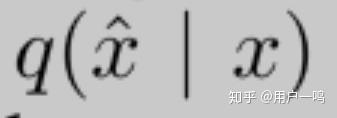
augmentation transformation from which one can draw augmented examples xˆ based on an original example x

